k-Means Clustering
K-means clustering is one of the simplest exchange methods. It may be used for larger datasets as it is rather quick if only a few cluster centers are involved. K-means clustering requires the number of clusters k to be specified by the user.
From a mathematical viewpoint k-means clustering is equivalent to an minimisation of the target function
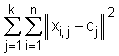
where is the distance between the datapoint i and the cluster center j.
Algorithm
1. Initialisation |
In order to initialize the algorithm, all k cluster centers have to be set to randomly selected data points. Alternatively, the first k data points in the dataset could be used. However, it is important that all initial cluster centers are located on different and unique positions in the p-dimensional space. The best results are achieved if the cluster centers are positioned in a way that the distances between the initial cluster centers are maximal. Each cluster center is assigned to a unique class number (1 to k).
|
2. Classification |
Find the closest cluster center for each data point in the dataset and assign the class number of this cluster center to it. |
3. Recalculate cluster centers |
Recalculate the positions of the cluster centers by averaging the coordinates of the class members beloging to the particular cluster. |
4. Iteration |
Repeat step 2 until the classification does not change any more. |
Disadvantages of the k-means algorithm
Apart from the simple and quick implementation the k-means procedure exhibits a few disadvantages which must not be overlooked:
- The final result of the clustering depends on the initial positions. It is thus recommended to repeat several trials using different start positions.
- It is possible that some clusters will be empty (no data points assigned to it)
- The classification is not invariant against scaling. As a remedy, the data should be standardised (if the data and the aim of the research allow it).
|